Artificial intelligence will play an important role in national and international
security in the years to come. As a result, the U.S. government is considering
how to control the diffusion of AI-related information and technologies.
Because general-purpose AI software, datasets, and algorithms are not
effective targets for controls, the attention naturally falls on the computer
hardware necessary to implement modern AI systems. The success of modern
AI techniques relies on computation on a scale unimaginable even a few
years ago. Training a leading AI algorithm can require a month of computing
time and cost $100 million. This enormous computational power is delivered
by computer chips that not only pack the maximum number of transistors—
basic computational devices that can be switched between on (1) and off (0)
states—but also are tailor-made to efficiently perform specific calculations
required by AI systems. Such leading-edge, specialized “AI chips” are
essential for cost-effectively implementing AI at scale; trying to deliver the
same AI application using older AI chips or general-purpose chips can cost
tens to thousands of times more. The fact that the complex supply chains
needed to produce leading-edge AI chips are concentrated in the United
States and a small number of allied democracies provides an opportunity for
export control policies.
This report presents the above story in detail. It explains how AI chips work,
why they have proliferated, and why they matter. It also shows why leading-edge chips are more cost-effective than older generations, and why chips
specialized for AI are more cost-effective than general-purpose chips. As part
of this story, the report surveys semiconductor industry and AI chip design
Center for Security and Emerging Technology | 4
trends shaping the evolution of chips in general and AI chips in particular. It
also presents a consolidated discussion of technical and economic trends that
result in the critical cost-effectiveness tradeoffs for AI applications.
In this paper, AI refers to cutting-edge computationally-intensive AI systems,
such as deep neural networks. DNNs are responsible for most recent AI
breakthroughs, like DeepMind’s AlphaGo, which beat the world champion
Go player. As suggested above, we use “AI chips” to refer to certain types of
computer chips that attain high efficiency and speed for AI-specific
calculations at the expense of low efficiency and speed for other
calculations.*
This paper focuses on AI chips and why they are essential for the
development and deployment of AI at scale. It does not focus on details of the
supply chain for such AI chips or the best targets within the supply chain for
export controls (CSET has published preliminary results on this topic1
).
Forthcoming CSET reports will analyze the semiconductor supply chain,
national competitiveness, the prospects of China's semiconductor industry for
supply chain localization, and policies the United States and its allies can
pursue to maintain their advantages in the production of AI chips,
recommending how this advantage can be utilized to ensure beneficial
development and adoption of AI technologies.
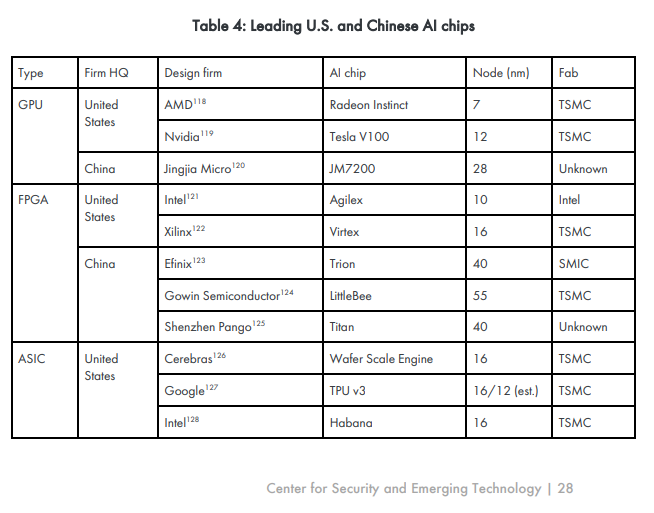